Our friend and colleague Harry Hatry has long insisted that data is far more valuable when it’s disaggregated. In a January 2022 paper for the Urban Institute which we were honored to co-author with him, he maintained that performance data is particularly useful when you “compare the outcome values broken out (disaggregated) by demographic characteristics (e.g., by age group, race/ethnicity, gender, education level, and location—such as neighborhood, state, or other geographical location).”
Much more recently, Michael Jacobson, director of Performance and Strategy at the King County Office of Performance Strategy and Budgets pointed us to an adage he once heard: "Aggregate to communicate and disaggregate to manage."
These lessons are brought into start relief by a just released report by the Census Bureau that will stop us from ever thinking that the median population of states is a truly meaningful figure. The title of the report gets right to the point: "New Census Bureau Visualization Shows Broad Variations in Age."
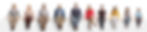
Consider Florida. Its median age in 2021 was 42.7, somewhat higher than next-door neighbor Georgia with a median age of 37.5. But does this mean that all of Florida is a place where retirees tend to go for low taxes and sunny weather? Not really, if you visit Leon County, with a population approaching 300,000. That county, home to the state capital, appropriately named for explorer Ponce de Leon, who is said to have sought the Fountain of Youth, has a median age of 32.1.
By wild contrast, Sumter County, Florida, has a median age of 68.3, the highest of any county in the country. No surprise there. Sumter County is effectively little more than the home of The Villages, a master-planned age-restricted community with 130,000 people and virtually no children.
Florida is not unusual, as the Census Bureau points out. Median ages ranged from county to county in practically every state. South Dakota, for example, had an extremely low median age of 23.0 in tiny Todd County, primarily home to Native Americans compared to 56.3 in Custer County.
These age ranges are of consequence for a number of reasons. For example, when states distribute finances to counties based on their total populations, it might be wise for them to take the individual counties’ median ages into account. Consider the funding that went out to counties to help them deal with Covid vaccinations, particularly in the early days of the pandemic. Given the greater likelihood of hospitalizations and deaths among older people, it would have made simple sense to look at these disaggregated figures and spend more in the counties with higher median ages.
Lumping people together into any monolith is often misleading, and age is only one example. A couple of months ago, Laura Zhang Choi, a Warren County school-board member testified to New Jersey legislators that the state would be well served by breaking down the component parts of New Jersey’s Asian American residents, according to an article in Verve Times.
She pointed the legislators to New York City as a powerful example, and according to the article she told the legislators that, “about 11% of city residents suffer from diabetes, and the rate among Asian Americans is roughly the same at 12%. But a deeper look showed an alarming figure for Indian Americans, nearly double the city average at 21%. That information disappeared when all Asian ethnicities were lumped together.”
The importance of disaggregation – for many other factors – was clearly spelled out in a recent podcast featuring Amy O’Hara, Research Professor in the Massive Data Institute at Georgetown’s McCourt School of Public Policy.
As she explained, “When we think about the way that our communities are reflected in data, the biggest regular data collection is a decennial census. Every 10 years information is pulled together about every single resident in the United States. And for that information, in order to do apportionment, you say, how many humans are there in the U.S. and that’s adequate for that purpose.
“But then, you really want to start breaking it down. What are the characteristics of these people? How many are male? How many are female? How many are old? How many are young? And you get these disaggregations of the data that were collected. The aggregate information is useful, but depending on what your policy question is, it’s not going to be useful enough.”
#PerformanceManagement #StateandLocalGovernment #Equity #PublicSectorData #CityandCountyManagement #PerformanceMeasurement #DisaggregatedData #MichaelJaccobson #KingCounty
#Disaggregation #UrbanInstitute #Covid #CensusBureau #PerformanceData #DataDisaggregation #KingCountyOfficeofPerformanceandStrategy
#DataVisualization #StateofFlorida #StateofGeorgia #StateofSouthDakota #StateofWashington #StateofNewJersey #NewYorkCity #StateofNewYork #StateandLocalGovernmentPerformanceMeasurement #McCourtSchoolofPublicPolicy