Building a large AI data model from scratch is prohibitively expensive for most organizations. But the release of AI foundation models, particularly large language models (LLM), has allowed organizations to take advantage of broader community investments in AI.
But there’s a catch: Agencies need to tweak and train these models to meet their specific objectives, maintain privacy and ensure regulatory compliance.
“These models are trained on a broad range of data, a wide understanding of language and concepts and images,” said John Dvorak, Chief Technology Officer for Red Hat North America Public Sector. “Fine-tuning is essential to maximizing the relevance of the model, the accuracy, the effectiveness for the specific use cases that you have.”
Understanding Foundation Models
Keep in mind that foundation models are purposely versatile and adaptable. Because they draw from a broad base of inputs, “they can be adapted for use across a wide variety of ranges and use cases,” Dvorak said.
But that strength is also a potential weakness. In their raw form, broad-based foundation models are not ideally suited to support the nuanced needs of government agencies, Dvorak said, which deal in “unique libraries or vocabularies, terminologies and processes, which aren’t necessarily captured or semantically linked in the model. They are also not tuned to address bias or handle concepts such as novel or emerging topics.”
Those models also may not be adept at protecting sensitive or private data, or may not act in accordance with agency regulations and collection authorities.
How MLOps Helps
By using their own data to adjust the foundation model, whether through fine-tuning or newer strategies such as Retrieval-Augmented Generation (RAG), agencies can drive more effective AI outputs. But any effort to maintain a model in production requires a secure, transparent and consistent process for making improvements over time.
This is where Machine Learning Operations (MLOps) comes into the picture. MLOps offers a streamlined approach to making iterative improvements to the model. “It’s taking that model through its life cycle: from data collection, to training that data, putting it into production and then monitoring — then, going back and doing it again,” Dvorak said.
Red Hat OpenShift AI provides a flexible and scalable platform for building AI-enabled applications. It includes all the elements of MLOps, empowering organizations to automate and simplify the iterative process of integrating machine learning models into software development processes, production rollout, monitoring, retraining and redeployment for continued accuracy.
With a flexible platform built on a scalable infrastructure, developers can hone the foundation models in support of AI applications that understand government’s highly specific subject matter, and align to its particular operational requirements.
“OpenShift AI can run on prem, in the cloud, or on the edge of your network. The platform is plug-and-play: It’s consistent, it’s flexible, and provides all the components” to train, fine-tune, serve and monitor models, Dvorak said.
This article appeared in our guide, “Gearing Up for AI.” To learn more about AI’s transformative impact in government and prospects for 2024, download the guide here:
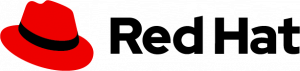
Leave a Reply
You must be logged in to post a comment.